The concept of DiCyano is illustrated in the above figure. Data from new monitoring technologies, e.g. sensors, satellite data (both in real-time and historical data), and species monitoring from case studies are collected, sorted, and integrated with other databases, e.g. weather data, historical data, climatic data, and routine sampling from both regional and national monitoring programs. This big dataset will be analyzed in a nexus for analysis on a computational platform where AI for big data analysis and machine learning (ML) play an important role. Big data analysis is intended to discover patterns and correlations among and between different parameters. By using the built-in program/algorithms, i.e. ML and process-based modeling, we will be able to predict changes in water quality parameters and explore potential risks. ML not only improves the model performance by increasing the accuracy of the predictions in a short-term perspective but is also able to better understand ecosystem properties for a long-term management purpose. Analysis results will be presented in a visualization platform based on the needs of the end-users, e.g. for early warning monitoring, risk assessment or mapping, bloom development and seasonal pattern, geographical risk mapping, potential risk prediction, and management strategies.
The basic package will be developed and tested in DiCyano with a series of analysis modules such as Basic Statistics, Time Series Analysis, Real-time Algae Monitoring, Species Dynamics, Bloom Early Warning, Water Quality Modelling, Future Climate Scenarios, and Mitigation Management. Those modules will serve as a cyanobacteria management toolbox, which can be used by each problem owner to design their customized solutions.
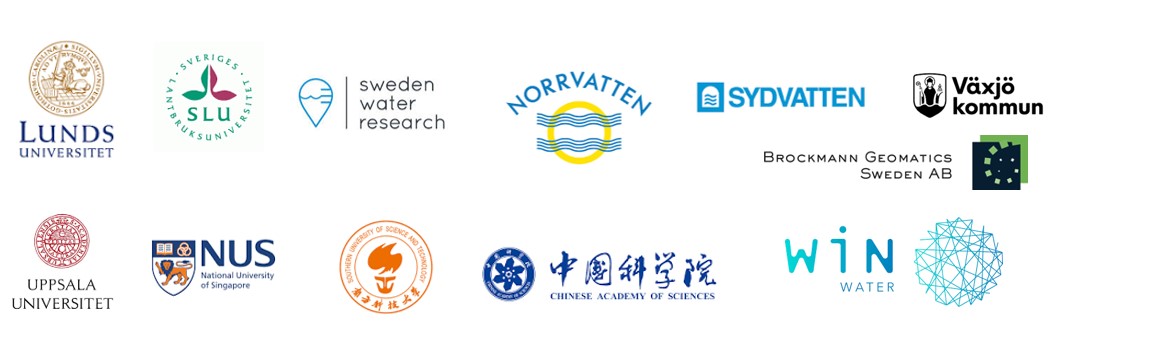